The Digital Ethics Compass:
Automation
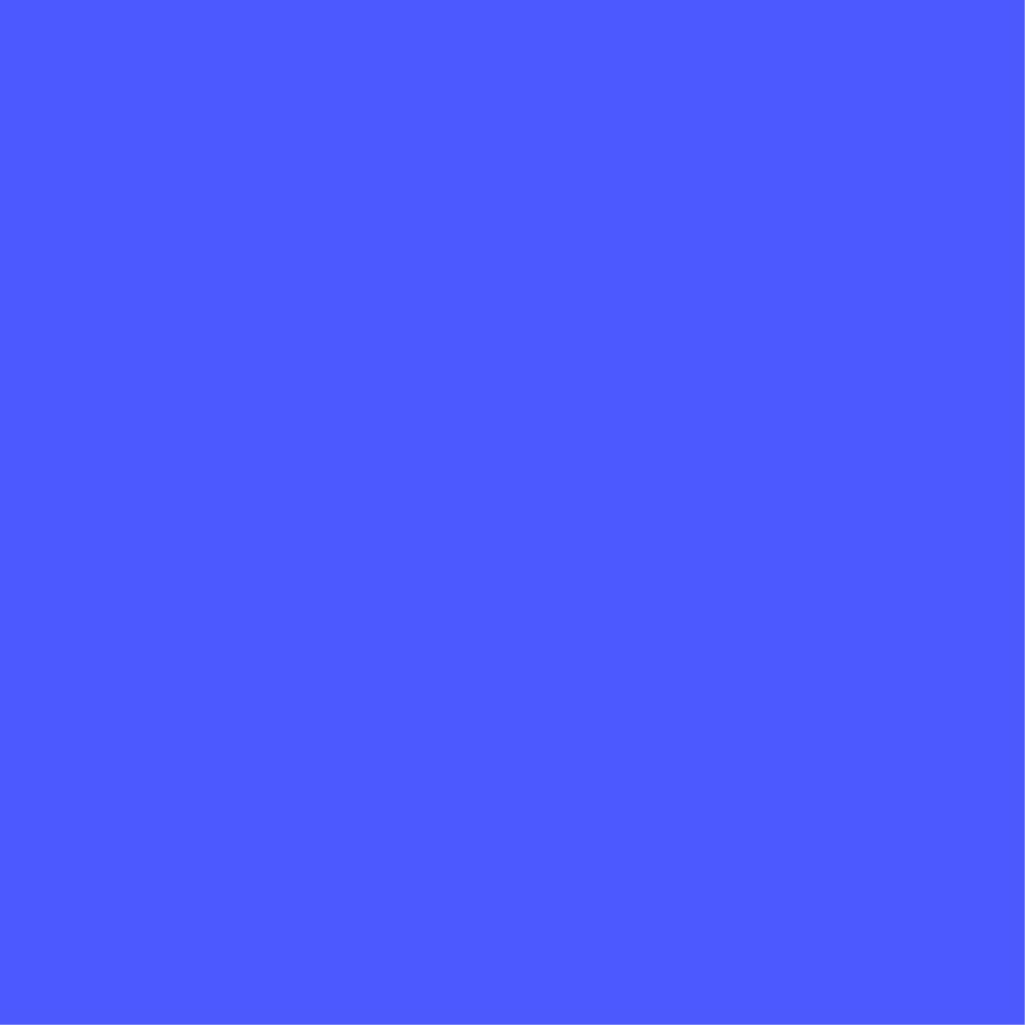
We can automate our digital solutions by using artificial intelligence and algorithms. Automation is often a good thing because machines can solve tasks faster and more accurately than humans, but on the other hand, machines also make mistakes that can be quite significant and have serious consequences for humans. It is your ethical choice whether you want to design automated solutions that help people or override people
01. Are your users aware that they are interacting with an automated solution?
Get smarter – what is it?
Get smarter – what is it?
Automated systems and artificial intelligence are getting increasingly better at communicating and acting like real people. They write in a grammatically correct manner and can speak so fluently that they can have conversations in ways that sound almost human. This phenomenon allows for new possibilities when setting up machines to handle communication tasks such as customer support, sales, secretarial functions, etc.
Ethical challenges arise when humans aren’t aware that they are communicating with an automated system. Automated systems have become good at simulating human behavior -but they are still not human, and therefore, they do things that are not human. They make unpredictable mistakes and are not able to understand or explain these mistakes themselves.
Humans have a need and a right to know when they are interacting with an automated system, especially if the AI makes decisions that have great importance to human life. There is a difference between an automatic e-mail confirming your online purchase and an automated refusal of the right to be with your child.
Recommendations
- Make it obvious to users that they are communicating with an automated system.
- Don’t design your automated system in a way that mimics human behavior (Chatting with a robot shouldn’t happen in the same interface as when chatting with a human).
- Make your automated systems more mechanical (preserve the robot voice and rigid robotic language).
The bad example
We all know Facebook’s newsfeed that is used to follow the lives of our friends and family. The newsfeed has become an automated system that makes calculations on our behalf and decides which updates we should see and which we should never see. When Facebook first released this automated version of the newsfeed, few users understood this update. Many users thought the newsfeed showed them all their connections’ updates and wondered why friends and family had nearly stopped existing on Facebook.
Facebook should have made it clear that its users were interacting with an automated system. It would have given users better control and understanding of their use of Facebook.
The good example
Many media companies use news robots to write simple articles about e.g., sports scores or stock quotes. In most cases, media companies draw attention to this. Jysk Fynske Medier, for example, writes: “Written by Jysk Fynske Medier’s article robot.”
02. Do your automated systems comply with legislation and human rights?
Get smarter – what is it?
Automated systems are increasingly being used for decision-making in cases that have an impact on people’s lives. The first ethical question you should ask yourself when designing an automated system is whether it makes decisions that respect human rights. A major problem with automated systems is that they can discriminate unfairly in decision-making processes. Discrimination may be ethically acceptable, but not if it’s based on factors such as gender, race, ethnicity, genetics, language, religion, political beliefs, disability, age or sexual orientation (not an exhaustive list).
Also, take care to ensure that your automated system does not harm children and that it respects the right to privacy and the right to freedom of expression.
Recommendations
- Strive to ensure that your development team has the widest diversity and openness towards the minorities’ use of your solution.
- Involve minorities and vulnerable target groups in your user research.
- Make sure that your automated system is verified by human rights experts.
The bad example
The Israeli artificial intelligence company Faception claims that it can analyze people’s faces and predict whether they are terrorists, academics, or highly intelligent. It is, however, doubtful whether the company is capable of this at all. There is a very high risk that their algorithms will come into conflict with fundamental human rights regarding discrimination. The algorithm will likely categorize people with an Arab/ Middle Eastern appearance as being terrorists.
The good example
Corti is a Danish company that has developed a machine-learning algorithm that listens to emergency calls. The algorithm can recognize patterns in conversations. In this case, an ambulance will arrive more quickly.
The algorithm from Corti has learned from old emergency calls data, which means that less common dialects are underrepresented. In the worst case, this could mean that the algorithm discriminates against these dialects and detects fewer cardiac arrests in areas where this dialect is present. Corti continuously checks the algorithms for bias, and in the case of the dialects, they have chosen to train the algorithm using data from several specific emergency calls with different dialects.
03. Does automation cause people to lose the abilities to do a job?
Get smarter – what is it?
You have probably tried driving a car with a GPS. You enter an address, and then the GPS tells you turn-by-turn where to go. The GPS has meant that many people have lost the ability to read a map and have become less able to find their way without it.
Is this loss of competence problematic? It’s a challenging ethical issue. But the fact is that the design of GPS’ causes people to lose skills. Could one have designed the GPS differently so that people don’t blindly follow the directions? Perhaps one way could be by having north always at the top and thus helping people develop an understanding of the geography they move around in.
In other words, could one use GPS’ to make people better instead of worse at finding their way around on their own?
Recommendations
- Think about the future: What would the world look like if all people lost that competence?
- Always try to design your systems in a way that doesn’t make people redundant but instead makes them better and happier at doing their jobs.
- Can you incorporate learning and competence development into your digital solutions to help people develop new skills?
The bad example
Mercedes and many other car companies have developed automatic systems that can parallel park a car without the driver having to touch the steering wheel or pedals. For many people, it is probably a great help. But it is also a feature that will mean that many people lose the ability to parallel park – an ability that can be useful for many years to come (before cars become fully self-driving).
Should Mercedes (and others) instead have designed automated systems that help their users become experts in parallel parking? For example, by letting the drivers do it themselves, but advising and guiding underway?
The good example
Gradescope is a tool that helps teachers mark school assignments. The teacher uploads the students’ work in the program, which automatically gives a grade. Then the program provides an overview of how the students are doing. Gradescope also allows the teacher to add comments and correct how students are marked, so it’s not solely up to the automation.
Gradescope has thereby ensured that the automation of marking assignments does not remove competencies from teachers. On the contrary, it provides them with benefits in the form of time saved from the manual marking of assignments and an automatic overview of students’ skills.
04. Is your automated system transparent, so the user can see the engine room?
Get smarter – what is it?
Automated systems are usually designed to make many quick decisions. These systems are generally not made for people to understand the decision-making system. In many cases, however, people must understand how an automated decision-making system works.
Algorithms can make mistakes and they can learn from “bad” data, which causes them to discriminate systematically. If you cannot open the engine bonnet to the automated system, you can’t find the errors and injustices either. Obviously, it’s only a few people that are actually able to open the bonnet and understand the algorithms behind it. But transparency can also mean making one’s algorithms accessible and open to experts and legislators who act on behalf of ordinary people.
If the algorithms contain trade secrets, you can invite independent experts inside for closed ethical reviews meaning you do not have to reveal these business secrets.
Recommendations
- Try to explain to the public how your automated systems make decisions.
- Make your algorithms open and accessible so that experts can “open the bonnet.”
- Invite experts to review your algorithms.
- Try to develop simple algorithms with humanly understandable logic.
- As much as possible, avoid black box systems where you do not even understand how the algorithms work.
The bad example
In 2018, Gladsaxe Municipality in Denmark developed an algorithm to identify families where the children were likely to be mistreated. The algorithm was based on a large amount of data and machine learning that calculated the probability of children being unhappy in their families. The project met with political opposition, however. For one, it was difficult to see how the algorithm decided that some children were more vulnerable than others. The lack of transparency caused citizens, politicians, and experts to lose confidence that the system was fair and sufficiently accurate. After a lot of tug of war, the project got shelved in 2019.
The good example
Facebook has been heavily criticized for allowing political actors to use the platform to target political ads at carefully selected segments. Using Facebook algorithms, actors can hit select groups with perfectly designed messages that the public will never witness. To address this issue, however, Facebook has made political ads open to the public. Anyone can view all the ads from a particular political actor, see how much they spend on advertising, and roughly who they are targeting with their ads.
The system is not aimed at ordinary people but rather at journalists who can use the system to monitor political parties and actors, typically during elections. The system is not perfect, but it’s an excellent example of implementing openness into an automated system.
05. Can your automated system explain itself?
Get smarter – what is it?
As citizens of democratic and free societies, we get explanations for the decisions that affect our lives. “You must pay a reminder fee BECAUSE you paid three days later than the agreed deadline” or “You must serve 30 days in prison BECAUSE you violated section 266 of the Penal Code”.
However, there are many automated systems that are designed in such a way that they are not able to provide such explanations. And especially machine learning systems can be bad at providing justifications that humans can understand. It can create ethical issues in cases where people expect to get an explanation for an algorithmic decision, but the system is unable to provide one.
As automated systems make more and more decisions, there is a need for the systems to be designed in such a way that they can provide an explanation for their decisions. However, it is largely an ethical trade-off when a system should be able to explain itself. Of course, this applies to decisions with far-reaching consequences for human life, but in practice, there will be many algorithmic decisions that do not need explanations because they are too trivial and mundane (for example, an algorithm that automatically turns off the light in a room).
Recommendations
- Always try to design algorithms that explain themselves while they’re being used.
- If possible, avoid black-box algorithms where an explanation is important.
- Always make sure through the digital design that users can request an explanation an algorithmic decision.
- Ultimately, one should always be able to get an explanation from a human being for an algorithmic decision.
- Make your algorithms open and accessible so that experts can “open the bonnet.”
- Invite experts to review your algorithms.
The bad example
Facebook has employees whose jobs are to view content that is reported by users or by algorithms. But due to the amount of content on the platform, the algorithms can take complete control in certain situations, for example, during the covid-19 pandemic, when most of Facebook’s employees have been home. It has turned out that the algorithms have been the cause of much content on the platform has been deleted, or profiles have been reported without proper reasoning. Craig Kelley (an MP in the UK) has found that his posts have been deleted without explanation. After complaints, Facebook could not explain why they had been deleted and denied responsibility, but it turns out that this happens for about 300,000 Facebook posts a day.
The good example
Rainbird is a company that provides algorithmic decision-making systems to financial companies. Rainbird helps banks and insurance companies respond to customer inquiries and detect fraud. Rainbird differs from other algorithmic systems because it always incorporates explanatory systems so that customer service employees can understand how the algorithms arrive at a decision. It means that they can give their customers human and understandable explanations if their account has been closed or if they have been denied a loan.
06. Are your algorithms prejudiced?
Get smarter – what is it?
Modern artificial intelligence and automated systems use machine learning and data from our society and world.
The ethical problems arise firstly when these data are bad and do not represent the real world, e.g. if one only trains a face recognition algorithm on white people. Secondly, biased algorithms can arise if they reflect a reality that is already biased. The algorithms simply automate existing biases such as racism or gender discrimination.
One talks about that the algorithms become biased – that is, skewed so that they do not represent reality or so that they discriminate in a way that is not desirable. It is important to understand that one can never remove biases completely, so the goal is not bias-free algorithms but rather algorithms where one knows their biases and where their biases are in line with widely accepted human biases.
Recommendations
- Verify that machine learning data represents all the stakeholders that may be affected by the algorithm.
- Avoid having your digital solution automate existing and unwanted biases.
- Regularly check your algorithms for bias and preferably use independent experts for this.
- Make sure to have diversity in your development team to raise awareness of unwanted bias.
The bad example
IBM, Microsoft, and Mevii have developed facial recognition software that they claim can identify people with 99% accuracy. But a study by MIT Media Lab found that this accuracy was only valid for facial recognition of white men. The accuracy decreased when identifying women and black people and mostly with black women, where the accuracy was 65%. It turned out that the datasets for this software were images of parliamentarians, which may explain the difference
in precision.
The good example
When companies write job advertisements, they can, through their use of language, discriminate against people based on gender, age, and social background. One example is writing in a language aimed at young people rather than older people. Textio is a program that uses artificial intelligence to identify this type of discrimination and guides companies towards more inclusive language use. Companies can thus use Textio when recruiting to ensure more diversity among employees.
07. Is there an unnecessarily high risk with your automated system?
Get smarter – what is it?
Automated decision-making can be divided into four categories with different ethical risks:
- The system makes precise decisions on issues with little to no consequences. Here there are no considerable risks.
- The system often makes erroneous decisions but on issues with little consequence. There is a case to be aware of risks here, but the effect of failure is minuscule.
- The system makes precise decisions, but the consequences of errors can be fatal. Here one should take great care to be sure that the automated system is robust and not biased. One should consider whether there should be used automation in the first place.
- The system often makes erroneous decisions on issues with fatal consequences. Here one should always avoid automation!
Recommendations
- Consider the consequences if your automated system fails.
- Work with worst-case scenarios.
- Be sure to monitor and evaluate the errors that your automated system makes.
- Involve independent experts and critics in the development of your automated solutions.
- Be careful with non-transparent black box systems used to automate critical functions.
The bad example
IBM’s artificial intelligence, Watson, is used to assist the examination, diagnosis, and treatment of patients. In 2018, it was discovered that Watson recommended incorrect and sometimes deadly medications to patients. This discovery resulted in Rigshospitalet in Denmark and Novo Nordisk abandoning their use of the technology.
The good example
When an app for digital coronavirus contact tracing in Denmark was in development in the spring of 2020, a broad societal and ethical debate arose about automated monitoring of people’s contact with each other. There was an important argument from the critics saying that the value of the contact tracing app did not measure up with the risk of the government gaining access to citizens’ location data. The risk of abuse was too big. The solution was to involve an expert group with an understanding of both ethics and technology, which resulted in a final solution where the risk of data misuse had been minimized by decentralizing data collection. The project could have been completely abandoned, but instead, it was decided to design a solution that minimized risks.
08. Is someone in the company ready to step in when automation fails?
Get smarter – what is it?
Artificial intelligence can seem superhuman and infallible because it can find patterns and make calculations on data otherwise incomprehensible to humans. But artificial intelligence also makes mistakes, and often these are surprisingly banal because artificial intelligence lacks the human understanding of how the world is connected.
It is therefore crucial that humans are never removed from automated decision-making systems. Firstly, you should make sure that you have someone constantly keeping an eye out for errors and irregularities in the system. Secondly, you should always ensure that your customers and users can get in touch with a human being if your automated system fails. The latter is also a requirement of the GDPR.
Recommendations
- Always be very careful if you remove people completely from your automated systems.
- Include a functionality in your digital solution that allows users to speak with a person.
- Make sure you have the right people on standby to intervene when automation fails.
- Be aware that working as a supervisor of automated systems can be monotonous, tedious, and uncomfortable.
The bad example
In 2011, the American t-shirt company, Solid Gold Bomb, developed an algorithm that could make funny slogans about the meme “Keep calm and carry on” for print on t-shirts. The system was fully automated, so the funny ones were put up for sale on Amazon without any people having checked the slogans. Solid Gold Bomb didn’t deem it necessary to observe the t-shirt slogans because the t-shirts would only be produced if people bought them.
Unfortunately, the algorithm began putting t-shirts up for sale with messages like “Keep calm and kill her” and “Keep calm and rape a lot.” The case exploded on social media, and the company went bankrupt due to bad publicity.
The good example
The Danish company Holo works with self-driving buses, which are currently driving around Copenhagen, Aalborg and Oslo. The buses can only get up to a speed of 15 km/h and have never been in any accidents, but Holo has still chosen to place a living person in all their buses, ready to intervene if the bus fails or if any other problems occur in the bus.
09. Is your automated system adaptable to changes?
Get smarter – what is it?
Automated systems are usually designed based on historical data. One automates actions and workflows that work today and reckons that they will also work in the future, but the world is constantly changing. Humans are changing preferences, attitudes, and patterns of action, which means that most automated systems that interact with humans will stop working if they are not continuously updated.
There can be several ethical consequences of static automatic systems. A self-driving car that does not get its algorithms updated with new maps will drive the wrong way. But static algorithms can also perpetuate unwanted prejudices that may have been acceptable in the past but have changed over time, for example, discrimination against women in the workplace.
Recommendations
- Always consider your automated system as a work-in-progress. It is never a finished product.
- Always train your machine learning systems on new data.
- Be aware that your automated system may need whole new types of data.
- Be sure to design curious artificial intelligence that tests and searches for changes and new patterns.
The bad example
In 2009, based on millions of users’ searches, Google Flu Trends managed to track down a flu epidemic in the United States two weeks faster than the U.S. Center for Disease Control and Prevention. Due to this, the expectation became that Google’s algorithm could predict precisely where and when an epidemic would strike in the future. But after 2009, Google’s predictions became inaccurate, and on several occasions, the service overestimated the scale of the outbreaks to such an extent that Google Flu Trends went extinct after five years.
The good example
When listening to music on Spotify, the company collects data about users’ taste in music used to generate music recommendation lists. It could develop into an echo chamber where the user listens to some music, gets recommended more of the same, and keeps listening to the same kind. But Spotify’s algorithms are good at testing the limits of their users’ musical taste, which means that users are constantly allowed to listen to new music and test their limits. The result is that the algorithm both helps push people’s music taste and follows when people change it over time (unless they are stuck on old eighties songs).
10. Can your automated system be hacked?
Get smarter – what is it?
Self-driving cars use artificial intelligence to interpret the sensory impressions they encounter through the car’s many sensors. The problem is that these sensory impressions can be hacked without breaking into the car but simply by changing the surrounding environment. For example, people have found that you can make self-driving cars overlook stop signs if you stick white tape in specific patterns on the sign. It is self-evident that you need to secure your digital solutions against traditional hacking, where people break into a system. But modern machine learning, which is based on real-world data, allows for entirely new ways of hacking.
Often it is not even malicious hacking but simply people wanting to make fun of the “robots.” For example, when people jump out in front of self- driving cars to test whether they will brake.
Recommendations
- Think in worst-case scenarios: There will always be someone trying to cheat your automated system.
- Consider how people will react to your automated system and take into account their reactions.
- Be aware that unethical systems will create more motivation for hacking and data manipulation.
The bad example
A Vietnamese IT security company has demonstrated that they can hack the facial recognition feature on an iPhone X. This is done by making a 3D printed “twin mask” for less than 2,000 Danish kroner (around 300 euros). So you do not have to be an expert in computers to break into an iPhone -all you need is a picture of your victim and access to a 3D printer.
The good example
Google is the world’s most important search engine, and it can be life or death for businesses whether they are at the top of Google’s search results or not. Therefore, of course, many try to figure out Google’s algorithms so that they can get higher up on the results page. Sometimes people cross the line and use methods that try to hack Google’s algorithm. It is also known as black hat search engine optimization.
Google is in a perpetual battle against these hackers, who do not break into Google’s systems but try to hack the data that Google uses to rank results. Google has so far won the battle against the black hats. But it is an eternal battle that requires thousands of dedicated employees who are constantly developing their algorithms.
The Digital Ethics Compass

Can’t get enough of design and innovation? We hear you. And we have you covered.
Sign up for our newsletter to get the latest from our world delivered straight to your inbox.
Sign up for the ddc newsletter